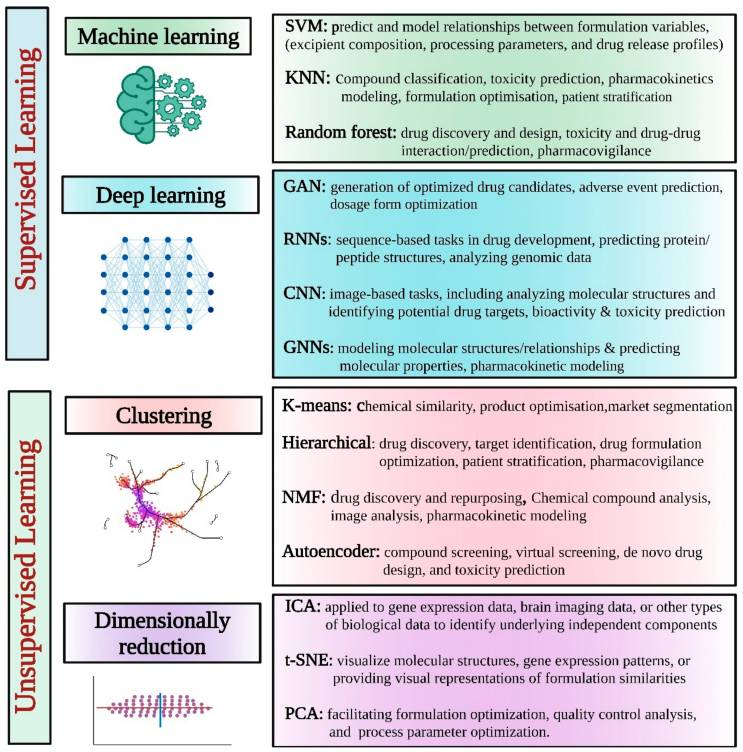
Artificial Intelligence in Pharmaceutical Technology & Drug Delivery
Introduction:
The intersection of artificial intelligence (AI) and pharmaceutical technology is reshaping the landscape of drug discovery, development, and delivery. As we stand at the forefront of a new era in healthcare, this article explores the transformative impact of AI on pharmaceutical technology and its crucial role in the innovative design of drug delivery systems.
AI in Drug Discovery:
Accelerating Drug Identification:
AI algorithms analyse vast datasets, expediting the identification of potential drug candidates. Machine learning models can predict how different compounds will interact with biological targets, significantly reducing the time and resources traditionally required for drug discovery.
Target Identification and Validation:
AI aids in the identification and validation of drug targets by analysing complex biological data. This enables researchers to pinpoint specific molecular targets involved in diseases, paving the way for more precise and effective therapeutic interventions.
Structure-Activity Relationship (SAR) modelling:
is a pivotal tool in drug discovery and design, aiming to elucidate the correlation between the chemical structure of a molecule and its biological activity. Through SAR modelling, researchers can analyse the subtle variations in molecular structures to predict how these changes impact the compound’s efficacy, potency, and safety. Leveraging computational techniques and statistical methods, SAR models help guide the rational design of new drug candidates by identifying essential structural features that influence the desired biological response. This iterative process enhances the efficiency of drug development, enabling scientists to make informed decisions about the synthesis and optimization of compounds, ultimately accelerating the journey from an initial concept to a potential therapeutic agent.
De Novo Drug Design:
AI-driven de novo drug design is revolutionizing the traditional approaches to drug discovery by harnessing the power of artificial intelligence. In this innovative method, machine learning algorithms analyse vast datasets of chemical and biological information to generate entirely novel molecular structures with therapeutic potential. These algorithms learn from existing knowledge of known drug-target interactions, molecular properties, and structural biology, allowing them to propose new compounds with optimized properties. AI de novo drug design expedites the identification of potential drug candidates, offering a systematic and data-driven approach to create molecules tailored to specific biological targets. This transformative technology holds promise for accelerating the drug discovery process, potentially leading to the development of novel and more effective medications for a wide range of diseases.
Optimization of Drug Candidates
AI algorithms can analyse and optimize drug candidates by considering various factors, including efficacy, safety, and pharmacokinetics. This helps researchers fine-tune therapeutic molecules to enhance their effectiveness while minimizing potential side effects.
Drug Repurposing
AI-based drug repurposing is a cutting-edge approach that leverages artificial intelligence to explore new therapeutic possibilities for existing drugs. By analysing vast datasets containing information on drug properties, molecular pathways, and disease characteristics, AI algorithms can identify potential matches between approved drugs and novel therapeutic targets. This innovative method accelerates the drug development process by repurposing existing medications for different medical conditions, saving time and resources. AI’s ability to uncover hidden relationships within complex biological data allows researchers to identify promising drug candidates for a variety of diseases, offering a more efficient and cost-effective strategy in the pursuit of novel treatments.
Toxicity Prediction
AI-based toxicity prediction is a transformative tool in drug development, employing sophisticated machine learning algorithms to forecast potential adverse effects of chemical compounds on biological systems. By analysing vast datasets encompassing known toxic and non-toxic substances, these algorithms discern patterns and relationships between molecular structures and toxicity outcomes. This predictive capability allows for early identification of potential safety concerns associated with drug candidates. AI-driven toxicity prediction not only expedites the drug development process but also aids in minimizing the risk of unforeseen toxicities during clinical trials. This innovative approach enhances the overall safety profile of pharmaceutical compounds, facilitating more informed decision-making in the pursuit of effective and secure therapeutic interventions.
AI in Pharmaceutical Formulation:
AI-driven algorithms optimise pharmaceutical formulations by predicting the ideal composition and structure. This accelerates the development of drug formulations with enhanced solubility, stability, and bioavailability, addressing challenges often encountered in traditional formulation processes.
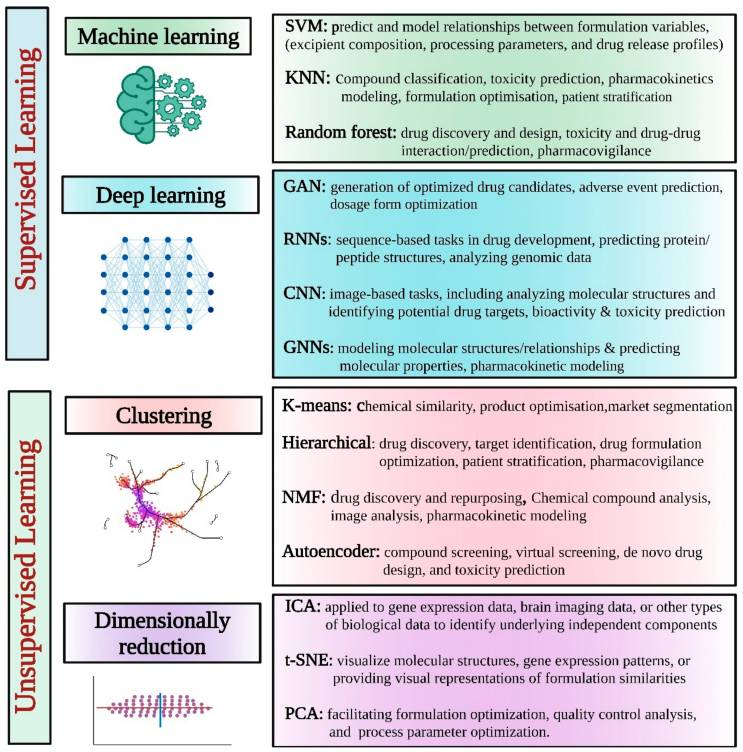
Personalized Medicine:
AI enables the design of personalized drug formulations tailored to individual patient profiles. By analysing genetic and clinical data, AI algorithms can predict the most effective dosage forms and delivery methods, optimizing treatment outcomes while minimizing side effects.
AI in Drug Delivery Design:
Nanotechnology and Smart Drug Delivery Systems:
AI plays a pivotal role in designing nanocarriers and smart drug delivery systems. By analysing patient-specific data, AI can develop nanoscale delivery vehicles that precisely target diseased cells, improving drug delivery efficiency and minimizing off-target effects.
Predictive Modeling for Pharmacokinetics:
AI-driven predictive models analyse complex pharmacokinetic data, providing insights into drug absorption, distribution, metabolism, and excretion. This information guides the design of drug delivery systems with optimal release profiles, ensuring therapeutic efficacy.
Challenges and Future Prospects:
Data Security and Ethical Considerations:
The integration of AI in pharmaceutical technology raises concerns about data security and privacy. As the industry embraces AI, it must also prioritize ethical considerations to safeguard patient information and maintain trust.
Continuous Learning and Adaptation:
AI systems require continuous learning and adaptation to evolving medical knowledge. Ongoing research is essential to refine algorithms, ensuring they keep pace with advancements in pharmaceutical sciences and technology.
Conclusion:
Artificial intelligence is a catalyst for unprecedented advancements in pharmaceutical technology and drug delivery design. By harnessing the power of AI in drug discovery, formulation, and delivery, the pharmaceutical industry is poised to revolutionize medicine, offering more targeted, efficient, and personalized treatments. As research and development in AI continue to unfold, the synergy between technology and healthcare promises a future where innovative solutions transform the way we approach disease management and patient care.